Generative AI Vs Predictive AI: Unveiling Future Innovations.
When it comes to AI, you’ve heard terms like Generative AI and Predictive AI, but what’s the difference?
Imagine two people sitting at the same table. One is a storyteller, and another is a fortune teller, both inspired by AI. Generative AI acts like a storyteller. It creates words, images, and music from nothing. It’s the magic that powers chatbots like ChatGpt and image creators like DALL·E, transforming your ideas into art, stories, or even entire worlds. On the other hand, Predictive AI acts as a fortune teller. By looking into the past, studying patterns, and trying to predict what might come next. It helps businesses forecast sales, detect fraud, and anticipate customer needs. One inspires your imagination, while the other provides clarity and foresight.
You may unlock incredible possibilities by combining your ideas with precision. When choosing between them, it’s not really about rivalry; it’s more about what you need at that moment. Both are game- changers, but you’ll find their strengths in different areas. So, whether creating an AI masterpiece or refining business strategies, knowing which type to use can make all the difference.
So, which one are you thinking of exploring first? Keep reading—you definitely don’t want to miss the rest of this exciting journey!
What is AI?
So, what exactly is AI ? Well, it’s basically like giving computers a brain, allowing them to learn, reason, and solve problems like humans do! Artificial intelligence, or AI for short, is the process of building intelligent machines capable of performing activities that normally need human intelligence. Think of things like voice assistants (hello, Siri!), self-driving cars, and even those recommendation algorithms that know you better than your best friend. It’s a super exciting field that’s changing the way we interact with technology every day. It’s making our lives easier and more interesting!
Generative AI Vs Predictive AI:
It’s really important for you to understand the differences between Generative AI and Predictive AI. Generative AI generates new data. You can create images, text, or even music with it. Predictive AI, on the other hand, uses previously collected data to predict what will happen next. This comparison will help you see their unique roles and make smart choices in your AI projects.
What Is Generative AI?
Generative AI is like that creative friend who can whip up art, music, or even text out of thin air! It uses advanced algorithms to understand patterns in data and then generate new content based on what it learns. Imagine feeding it a bunch of paintings, and it can create a brand new piece that feels just as authentic. It’s not just about copying what’s out there. It’s about pushing creativity and exploration in new ways. So, whether you’re looking to brainstorm ideas or want to see some cool new art, generative AI has got you covered!
Concept and Definition
Generative AI is among the most incredible advancements in the tech world today! At its core, it refers to artificial intelligence systems that use advanced algorithms to generate new data.
Think of it like having a super-smart assistant that can whip up original pieces based on patterns and styles it has absorbed from a massive pool of information. This technology is revolutionizing industries by enabling new forms of creativity and efficiency, allowing artists, writers, and designers to push the boundaries of their work. From generating realistic images of people who don’t exist to drafting entire articles in seconds. Generative AI is reshaping how we interact with digital content!
Applications And Use Cases
Generative AI has many applications. It is used in various industries for different purposes.
- Content Creation & Marketing: Generative AI can write articles, stories, and reports. It helps content creators produce more in less time. It also has the amazing ability to create innovative and engaging marketing content, like blog posts or social media updates.
- Art and Design: Artists use generative AI to create unique pieces. Designers can generate new product ideas and concepts.
- Music Production: Musicians use AI to compose new tunes. It can generate melodies, harmonies, and even full songs.
- Healthcare: In healthcare, AI generates synthetic medical data. This helps in training algorithms without compromising patient privacy. Also, generative models in the pharma industry can design unique molecules for new drugs.
- Visual Media & Gaming: Generative AI can produce realistic simulation scenarios for training. These virtual environments provide a secure, cost-effective way to teach professionals tricky tasks. Additionally, Game developers use Generative AI to create new levels and characters. It makes games more dynamic and engaging.
- Coding and Debug: Generative AI coding help can make software development 45% faster. It can build programs or code based on specific needs.
Generative AI Applications
What Is Predictive AI?
Maybe you’ve heard about Predictive AI and are wondering, “”What the heck is it? Let me explain it to you, then! Predictive AI is an excellent branch of artificial intelligence that predicts the future based on past data by utilizing statistical algorithms, data, and machine learning.
Imagine having a crystal ball that can predict customer behavior, market trends, or weather! Predictive AI can help businesses make smarter decisions, boost efficiency, and personalize customer experiences by analyzing patterns and trends. It’s like having an intelligent assistant who always knows what’s coming next.
Concept And Definition
Predictive AI is all about analyzing past data to predict what might happen in the future. These algorithms learn from previous information. You find patterns and trends in the data. This allows you to precisely forecast future events. Critical components of Predictive AI include:
- Data Collection
- Data Analysis
- Algorithm Development
- Model Training
For instance, a retail store might use Predictive AI to forecast sales trends. This helps in inventory management and strategic planning.
Applications And Use Cases
Predictive AI is used in various industries. Here are some common applications:
- Healthcare risk assessment: Predictive AI models in healthcare can estimate the chance of patient readmissions or disease outbreaks. This strategy allows for optimal resource allocation and preventative measures.
- Retail Demand Forecasting: Two of the many difficulties supply chain managers face are Logistics interruptions and supplier unpredictability. According to a survey by Gartner, 60% of chief supply chain executives are expected to make decisions more quickly, accurately, and consistently. Hence, Predictive AI enables retailers to manage their inventory better. They can estimate customer demand for particular products. Also, through Predictive AI Demand forecasting, overstocking and stockouts decrease.
- Fraud detection in finance: Predictive AI algorithms can spot fraudulent transactions. Finding problems early helps businesses avoid financial loss. Also, predictive AI aids in stock market forecasts and assessing risks. This support improves the decision-making process and fosters business growth.
- Customer Churn Prediction: In subscription services, predictive models can help spot which customers are most likely to leave. Businesses can implement retention strategies for these customers.
- Predicting Energy Consumption: Utility companies can use predictive AI to see how energy usage patterns might look in the future. This is great for improving the grid and encouraging people to save energy.
- Predictive Maintenance in Manufacturing: AI models can predict when machinery might fail. This allows for maintenance before an expensive breakdown occurs. This increases the smoothness of things running and helps the machinery last longer.
- Agricultural Weather Forecasts: Predictive AI models can help forecast weather conditions, which is super helpful for farmers when making decisions about irrigation and harvesting.
- Forecasting Natural Disasters: AI can analyze environmental data to predict the probability of natural disasters like hurricanes and earthquakes. Early warnings can help us prepare, which might save lives and reduce property damage.
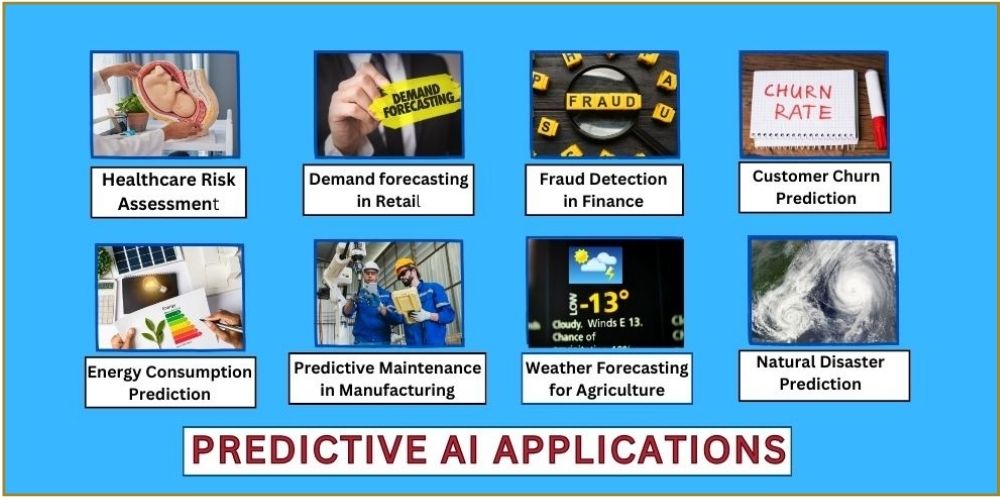
You can reduce risks and enhance your operations with the help of predictive AI. It can evaluate large amounts of data and provide you with insightful information. Your insights drive strategic choices and boost productivity.
Core Technologies
You need to understand the core technologies behind Generative AI vs Predictive AI. The technologies you see here shape how these AI systems function and solve problems. This section will break down the core technologies into two primary areas: Algorithms, models, and Data Requirements.
Algorithms And Models
Generative AI and Predictive AI rely on different algorithms and models.
Generative AI uses algorithms to create new content. This includes text, images, and sounds. Common models include:
- Generative Adversarial Networks (GANs): Two neural networks compete against each other to produce realistic outputs.
- Variational Autoencoders (VAEs): These models learn to encode input data in a compressed manner before decoding it back into its original format.
- Transformers: Advanced models like GPT-3 generate human-like text based on input prompts.
Predictive AI, on the other hand, focuses on forecasting outcomes. It uses algorithms like:
- Regression Models: These models predict continuous outcomes, such as sales forecasts.
- Classification Algorithms: They categorize data into predefined classes, like spam detection.
- Time Series Analysis: These models predict future values based on past data trends.
Data Requirements
Data is essential for both Generative and Predictive AI.
Generative AI requires large datasets to learn and create new content. The quality and diversity of the data impact the output. Fundamental data types include:
- Text Data: For natural language processing tasks.
- Image Data: For generating realistic images.
- Audio Data: This is used to create new sounds and music.
Predictive AI depends on historical data to make accurate predictions. This data should be clean and well-structured. Important data types include:
- Numerical Data: For regression and time series models.
- Categorical Data: For classification tasks.
- Time-Stamped Data: This is used to analyze trends and patterns over time.
Both AI types need substantial, relevant data to perform well. They must be trained on diverse, high-quality datasets to produce accurate results.
Generative AI VS Predictive AI Implementation: Success Stories
Generative AI:
This AI technology has been widely adopted across various industries, helping companies enhance creativity, efficiency, and customer experience. Here are some real-world scenarios and companies that have successfully implemented generative AI:
Entertainment & Media:
Company: OpenAI (via ChatGpt)
Use Case: Content Creation
ChatGpt OpenAI’s has been used by content creators to generate articles, stories, scripts, and even music. The AI’s ability to create text-based content has reduced writers’ time to develop ideas and drafts. Media companies and bloggers use it to assist with copywriting and brainstorming.
Success: Many digital marketing agencies, media outlets, and individual creators have incorporated ChatGpt into their workflows, increasing productivity and creativity.
Healthcare:
Company: Insilico Medicine
Use Case: Drug Discovery and Development
Insilico Medicine uses generative AI for drug discovery. The company has developed an AI model to generate novel molecular structures that could lead to effective new drugs. They use AI to design molecules with specific properties to treat diseases such as cancer.
Success: Back in 2020, Insilico Medicine identified a really promising drug candidate for idiopathic pulmonary fibrosis, and they went ahead with its development and got it into clinical trials. This really made the company shine in the crowded biotech sector.
Fashion & Design:
Company: Stitch Fix
Use Case: Personalized Fashion Design
Stitch Fix uses generative AI to create personalized clothing designs and suggest items that users might love. The platform’s AI looks at what users like, their styles, and body types to recommend clothing that suits their needs and tastes.
Success: Stitch Fix has enhanced its customer engagement and sales by offering hyper-personalized shopping experiences, leading to high customer retention and growth.
Video Games:
Company: Ubisoft
Use Case: Procedural Content Generation
Ubisoft has been using generative AI to craft huge and engaging virtual worlds in their video games. One cool application is making dynamic worlds where the environment and challenges pop up in real time, all based on what players do and how they interact.
Success: This use of generative AI has enhanced player experiences by making game worlds more engaging, unpredictable, and personalized, leading to better retention rates for Ubisoft games.
Predictive AI:
Predictive AI is successfully used across various industries to forecast future outcomes, optimize decision-making, and improve efficiency. Here are some real-world scenarios and companies that have harnessed predictive AI to drive success:
Retail:
Company: Walmart
Use Case: Inventory Management & Demand Forecasting
Walmart uses predictive AI to forecast demand, optimize inventory levels, and improve supply chain management. The AI examines past sales data, weather patterns, holidays, and local trends to predict customer needs and assist Walmart in keeping stock levels in line with demand.
Success: This predictive AI model has helped Walmart reduce stock outs and overstocking, ensuring that products are available when customers need them, leading to improved sales and customer satisfaction.
Healthcare:
Company: McKinsey & Company (McKinsey’s Healthcare Practice)
Use Case: Patient Outcomes & Disease Prediction
McKinsey’s healthcare practice applies predictive AI to improve patient care by predicting the likelihood of certain diseases, outcomes, and hospital readmissions. The AI predicts potential risks by analyzing patient history, lifestyle data, and medical records and enables doctors to take preventive actions.
Success: Predictive AI has helped healthcare providers improve patient outcomes, reduce readmission rates, and optimize resource allocation, which leads to better care and cost savings.
Banking & Finance:
Company: American Express
Use Case: Fraud Detection & Credit Scoring
American Express uses predictive AI to detect fraudulent transactions and assess credit risk. The AI analyzes transaction history, spending patterns, and customer behavior to predict whether a transaction is fraudulent and to evaluate the risk of lending to an individual or business.
Success: This use of predictive AI has reduced the number of fraudulent transactions, minimized financial risk, and improved customer trust, contributing to higher customer retention and profitability.
Manufacturing:
Company: General Electric (GE)
Use Case: Predictive Maintenance
GE uses predictive AI to maintain industrial machinery and equipment. The AI analyzes sensor data from machinery to predict potential failures or maintenance needs before they occur. This allows GE to address issues proactively and minimize downtime.
Success: By reducing unexpected breakdowns and extending equipment lifespans, GE has improved operational efficiency and reduced maintenance costs, resulting in substantial savings and higher uptime.
Generative AI vs Predictive AI (Strengths And Limitations)
Generative AI and Predictive AI both have unique strengths and limitations. Understanding these can help you choose the suitable AI model for specific needs. Below are detailed pros and cons for each type of AI.
Generative AI Pros And Cons
Generative AI creates new content. It can generate text, images, music, and more, making it versatile in many applications.
Strengths | Limitations |
---|---|
|
|
Generative AI’s biggest strength is its ability to create content. Yet, it has high computational requirements and varying quality.
Predictive Ai Pros And Cons
Predictive AI forecasts outcomes based on data. It is used in various fields like finance, healthcare, and marketing.
Strengths | Limitations |
---|---|
|
|
Predictive AI excels in making accurate forecasts. It helps in decision-making but relies heavily on data quality.
Generative AI vs Predictive AI (Future Trends)
The future of AI is always changing. Generative AI and Predictive AI are leading the way. Getting a grasp on future trends can really help you and your business adapt.
Evolving Capabilities
Generative AI is becoming more advanced. It can now create high-quality content, art, and even music. These capabilities will continue to grow.
Predictive AI is also advancing. It uses data to forecast future events. This helps in sectors like finance, healthcare, and retail.
Improvements in both types of AI are going to lead to smarter systems in the future. Systems that understand and adjust to complicated situations.
Generative AI vs Predictive AI Market Projections
Have you noticed the rapid growth of the AI technology market? Really, it’s taking off! The table below shows projected growth for Generative AI and Predictive AI.
Year | Generative AI Market Size (in billion USD) | Predictive AI Market Size (in billion USD) |
---|---|---|
2023 | 5 | 10 |
2025 | 12 | 18 |
2030 | 30 | 40 |
The numbers show significant growth. Generative AI is expected to see a six-fold increase by 2030. Predictive AI is projected to quadruple during the same period.
- Both AI types will see rapid growth.
- Generative AI will excel in content creation.
- Predictive AI will dominate data forecasting.
Businesses should keep an eye on these trends. Adapting to new AI capabilities can provide a competitive edge.
Conclusion
In summary, our comparison of Generative AI and Predictive AI shows that each technology has unique benefits and capabilities. Generative AI excels at creating new content, like text, images, and music. It pushes the limits of creativity and innovation. On the other hand, Predictive AI analyzes data to forecast trends and behaviors. It is invaluable for businesses seeking to make informed decisions. While they serve different purposes, the power of combining these two approaches can lead to groundbreaking advancements in various fields. So, whether you’re a creative seeking inspiration or a data-driven decision-maker, understanding the strengths of each can help you harness their potential in your projects!
Frequently Asked Questions
How Does Generative Ai Work?
Generative AI uses algorithms to produce new data similar to the training data.
How Does Predictive Ai Work?
Predictive AI uses statistical models to predict future outcomes from past data.
What Are Examples Of Generative Ai?
Examples include creating art, writing text, and generating music.
What Are Examples Of Predictive Ai?
Examples include weather forecasting, stock market predictions, and recommendation systems.
Can Generative Ai Create Realistic Images?
Yes, Generative AI can create highly realistic images from scratch.
Can Predictive Ai Improve Over Time?
Yes, Predictive AI improves as it learns from new data.
Which Industries Use Generative Ai?
Industries like entertainment, advertising, and design use Generative AI.
Which Industries Use Predictive Ai?
Industries like finance, healthcare, and marketing use Predictive AI.
Loved this post! Clear, concise, and useful.
Useful article. Great job on this post.
Микрозаймы без отказа: что важно знать перед подачей заявки?
микрозаймы онлайн https://www.dengikg.ru/ .
Well-written.
Very informative article. I learned a lot from it.
The way you structured this article kept me engaged from start to finish. Fantastic work!
I admire your storytelling style—it makes the article not just informative but also enjoyable to read.
Impressive and engaging. Thanks for sharing.
Insightful and engaging. Your insights are spot-on.